What is the SAMueL-2 project?
The SAMueL-2 project team developed modelling tools to understand the variation in the use of clot-busting drugs in hospitals, and support services to optimise their use in order to maximise patient outcomes.
What is the background?
Stroke is a leading cause of death and disability with over 85,000 people hospitalised in the UK each year. One way of treating stroke and preventing disability is to give a patient medication to break down blood clots. This is called thrombolysis.
However, thrombolysis is not suitable for all patients and can be risky. For thrombolysis to be useful it needs to be given as soon after the stroke as possible. Use of thrombolysis varies hugely, even for patients with similar treatment pathways and with similar characteristics; some hospitals rarely use it while some use it in a quarter of stroke patients. The speed of giving thrombolysis also varies, with some hospitals taking an average of 90 minutes and others taking less than 40 minutes to administer the drug.
Following the SAMueL1 project, we successfully gained funding to develop this research further.
How are researchers trying to help?
Building on our work from SAMueL-1 we will continue developing our clinical pathway and machine learning computer models, to investigate the variation in thrombolysis use in hospitals. In particular we will use ‘Explainable Machine Learning’ to understand what factors affect the decision to give thrombolysis or not at different hospitals. We will also expand the machine learning to predict outcome, and to understand what patient features most influence outcomes after stroke, with and without thrombolysis.
We will also be focussing on co-production of project outputs with clinicians to support the use of our tools for quality improvement. We will use interviews and workshops to continually improve our outputs and ensure they work for clinicians.
What has happened so far?
Where in SAMueL-1, hospitals were anonymised, we are now looking at individual hospitals’ data so that we can offer them advice about how to increase thrombolysis use. This will be delivered through the ’Thrombolysis in Acute Stroke Collaborative’ (TASC) group, which is being set up by NHS England.
We be publishing work comparing the clinical effectiveness of thrombolysis in practice, and comparing that to the results of the clinical trials.
We have launched a web app to allow hospitals to compare their decision making (which patients they would or would not treat with thrombolysis). We plan to expand the app so that hospitals can explore the likely effect of changing aspects of care. For instance, earlier access to scans for people with suspected stroke.
What happens next?
Methods used in this study to analyse the national stroke audit data may be transferable to other national clinical audits such as maternity care. Future studies could explore how machine learning could predict outcomes of thrombolysis for individual people, including the negative effects. This could be useful as some doctors were concerned that increased thrombolysis use could cause harm.
PenARC Staff
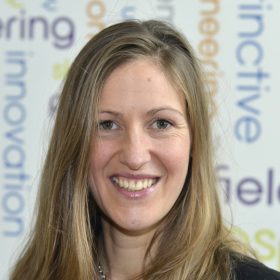
Kerry Pearn
Research Fellow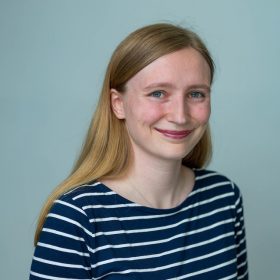
Dr Anna Laws
Research Associate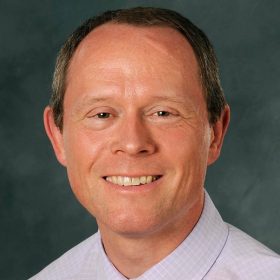
Professor Martin James
Consultant Stroke Physician and Honorary Clinical Professor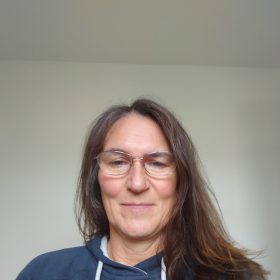
Prof Julia Frost
Associate Professor in Health Services Research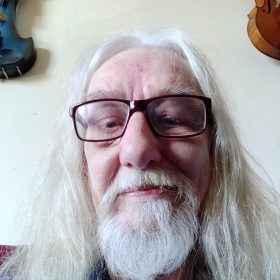
Leon Farmer
PenPEG Member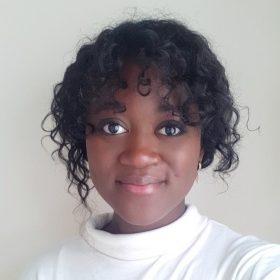
Lauren Asare
Graduate Research Assistant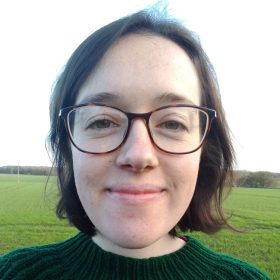